The world is moving so fast in AI. Taking the famous comparison from traditional Internet website to Social Media, and then AI, we can find the the pace of adoption is speedy.
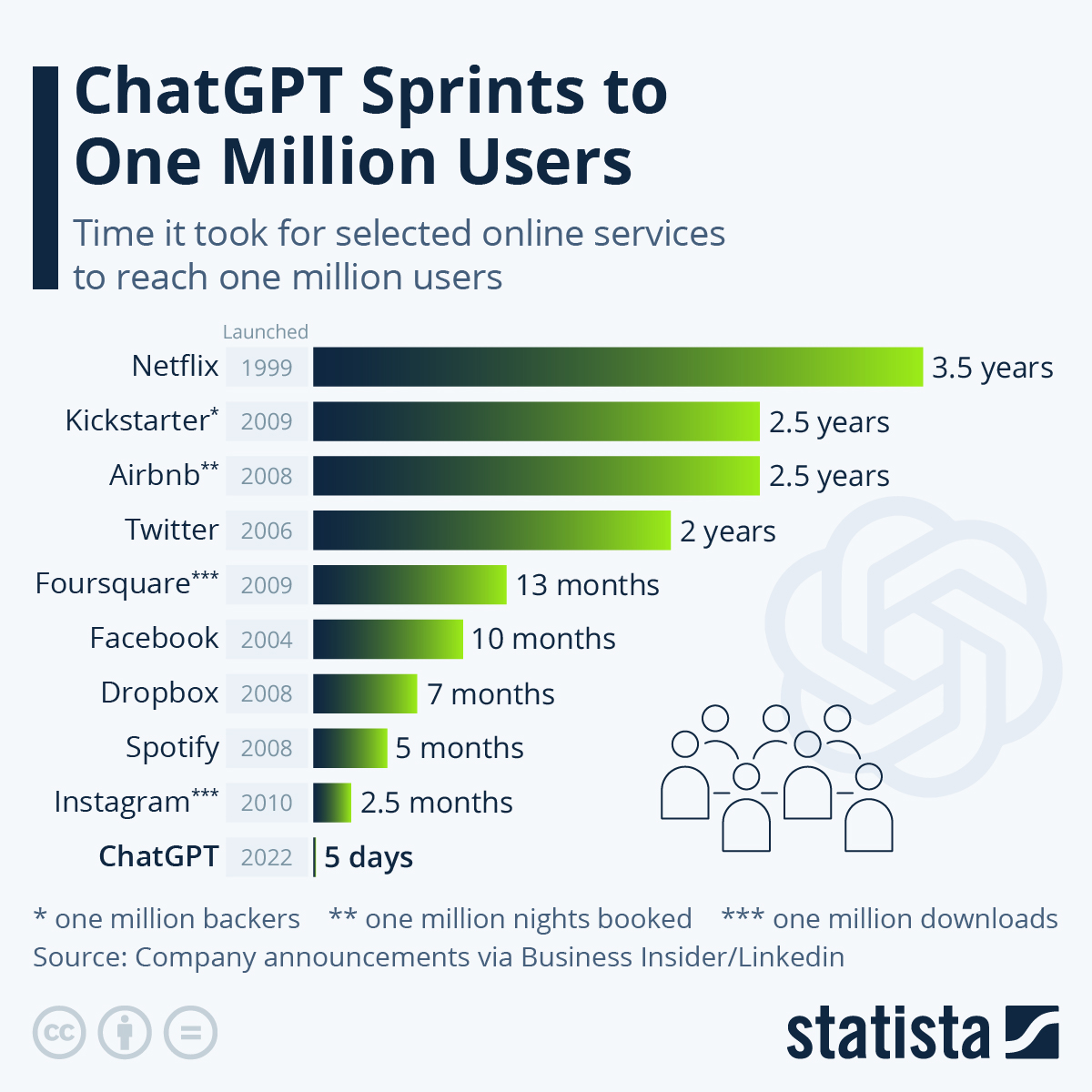
It comes with a cost of fast learning, as a Venture Capital investor, we have to catch up with all latest development. We need to check on latest technology, especially in Open Source world, and closely monitor the community around the AI eco-system, for example, how tools can help creators generate better prompt.
I was also shocked that even middle schoolers learned to use those AI tools to help themselves. I even had a lengthy discussion with my son, who is an 8th grader. The discussion has been primarily focused on what’s next for their generation. We reviewed the development of painting after the invention of the camera technology. The art evolved to be more representative of ideas rather than emulation of reality. The same thing will likely happen with the vast advancement of AI. Young generation will need to have better skills to find questions, define solutions, not so much about specific techniques.
There are various facets of AI impact to our daily lives. As an investor, especially a small VC firm, we need to find our niche interests in verticals. We have long decided that Large Models won’t be a battle ground that we can step in. We want to find some markets that is so fundamental (meaning large TAM) and yet unique. I love a graph shared to us by our friend Prof QF Xia from Mass, it shows with our current computational structure, we will soon run out of power if we don’t have a substantial change in our architecture.
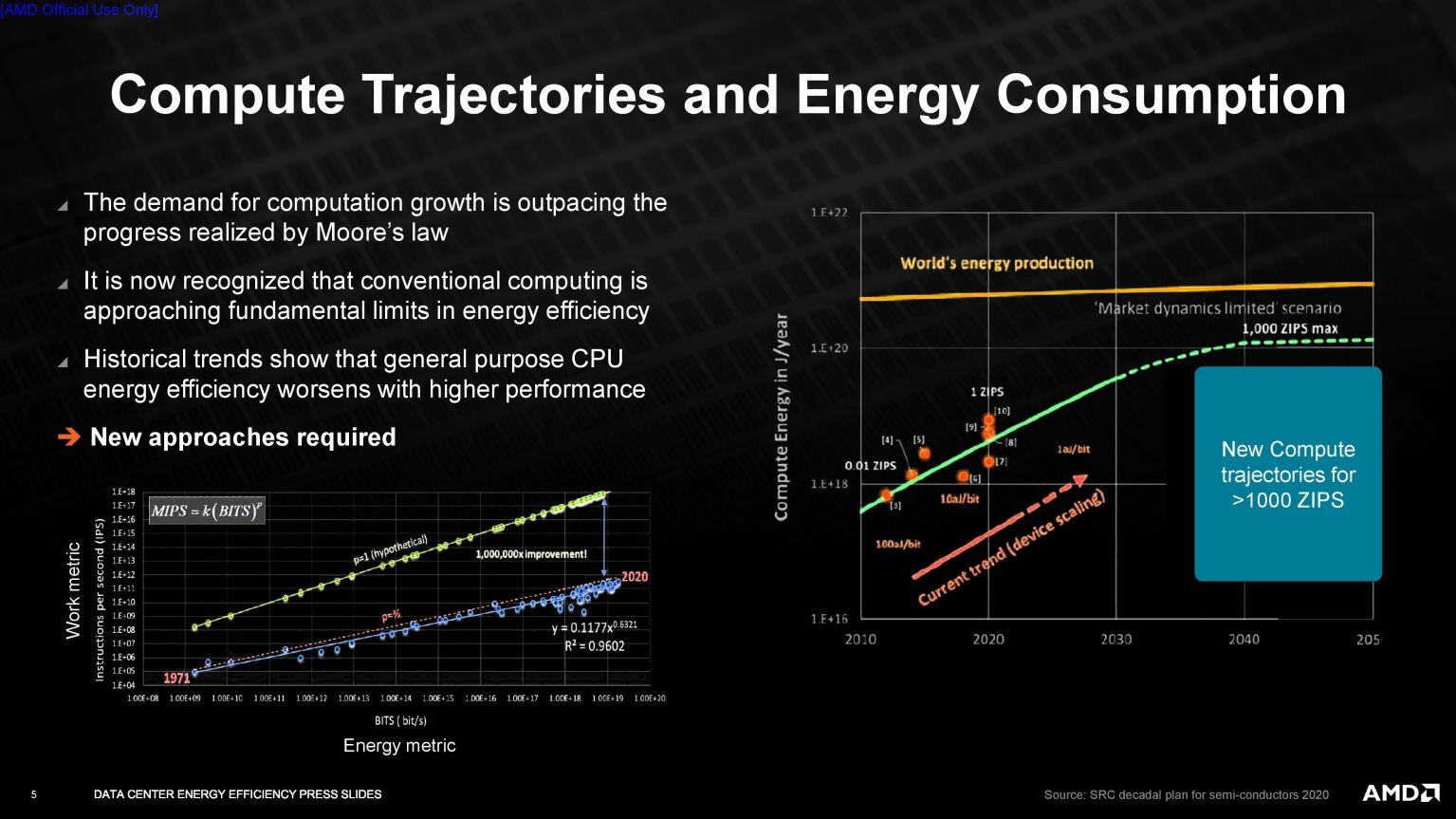
There are clearly two ways to look at this problem. One is from the cloud angle. With major players including Nvidia, AMD and Intel, the process node is getting more aggressive, and integration with faster HBM and faster connectivity is inevitable. There probably won’t be significant challenger on the horizon. On the other hand, the edge computing presents a bigger opportunity, after all, most data are collected at edge and the inference process need to happen at edge. In a very long time, we should still have most training done at cloud, but the model deployment at edge. The power efficiency at edge will be a key part.
We also need to take look at the AI application side, with most recent dispute on Google’s latest Gemini’s bias had taught us at least one thing. The data fed into the model training has consequences. The same logic can apply to other AI models. The quality of data became an essential part of the AI application quality. We must make deep study on the data quality in our investment process.
The overall demands for AI are faster, cheaper and reliable. Just like the parameters platforms use to build the leaderboards for various models, we have to dive into those areas to find opportunities. It is a reverse thinking process in Betawave.
As previous startup founders, we are also keen to understand better the “X” industries that can be revolutionized by AI adoption. I had a pick-and-place robot startup company over ten years ago. Reflecting on the product market fit of this company and the timing of the inflection point of that market, we believe AI will disrupt the automation industry much faster than we thought. This is an area we want to explore more. Not necessarily generic robotics, but more related to specific tasks that can be quickly deployed in a manufacturing environment to make productivity impact sooner.
With all that, I would also encourage our investors and our readers to try out different things in this AI hype. Not just Chats, Text to image or Text to video, but the platform AI can run on, and the adoption tools. Maybe LM studio is a good start. I would say Hugging face is also a website we all should visit on daily basis.